Neste tutorial, veremos como realizar o reconhecimento de objetos com Python usando uma rede neural pré-treinada usando aprendizado profundo.
Vimos num tutorial anterior como reconhecer formas simples utilizando a visão por computador. Este método só funciona para certas formas simples predefinidas. Se quiser reconhecer uma maior variedade de objectos, a forma mais fácil é utilizar a inteligência artificial.
Hardware
- Um computador com uma instalação Python3
- Uma câmara
Princípio
A inteligência artificial é um domínio da informática em que o próprio programa aprende a realizar determinadas tarefas. O reconhecimento visual, em particular. Neste tutorial, vamos utilizar uma rede neural treinada para reconhecer formas específicas.
São necessários muitos dados para poder treinar corretamente uma rede neural. Foi demonstrado que a aprendizagem é mais rápida numa rede neuronal treinada para outra coisa. Por exemplo, uma rede neuronal treinada para reconhecer cães será treinada mais facilmente para reconhecer gatos.
Configurar o Python
Caso contrário, pode descarregar e instalar o Python 3
Pode então instalar as bibliotecas OpenCV, numpy e imutils necessárias.
pip3 install opencv-python numpy imutils
ou
python3 -m pip install opencv-python numpy imutils
- ficheiro prototxt : https://github.com/nikmart/pi-object-detection/blob/master/MobileNetSSD_deploy.prototxt.txt
- ficheiro caffemodel : https://github.com/nikmart/pi-object-detection/blob/master/MobileNetSSD_deploy.caffemodel
Coloque os ficheiros do modelo numa pasta e crie o ficheiro ObjectRecognition.py

Script Python para reconhecimento de objectos
Em primeiro lugar, criamos um fluxo de vídeo (vs) utilizando a biblioteca imutils, que irá obter as imagens da câmara.
vs = VideoStream(src=0, resolution=(1600, 1200)).start()
Inicializamos uma rede neural com os parâmetros da ModelNet-SSD (net) utilizando a biblioteca OpenCV.
net = cv2.dnn.readNetFromCaffe(args["prototxt"], args["model"])
Em seguida, criaremos um ciclo que, em cada iteração, lerá a imagem da câmara e passá-la-á para a entrada da rede neural para deteção e reconhecimento de objectos.
while True:
# Get video stream. max width 800 pixels
frame = vs.read()
blob = cv2.dnn.blobFromImage(cv2.resize(frame, (300, 300)), 0.007843, (300, 300), 127.5)
# Feed input to neural network
net.setInput(blob)
detections = net.forward()
Por fim, o código apresenta a caixa de deteção e a probabilidade de reconhecimento na imagem.
label = "{}: {:.2f}%".format(CLASSES[idx],confidence * 100)
cv2.rectangle(frame, (startX, startY), (endX, endY),COLORS[idx], 2)
y = startY - 15 if startY - 15 > 15 else startY + 15
cv2.putText(frame, label, (startX, y),cv2.FONT_HERSHEY_SIMPLEX, 0.5, COLORS[idx], 2)
#!/usr/bin/env python # -*- coding: utf-8 -*- # # ObjectRecognition.py # Description: # Use ModelNet-SSD model to detect objects # # www.aranacorp.com # import packages import sys from imutils.video import VideoStream from imutils.video import FPS import numpy as np import argparse import imutils import time import cv2 # Arguments construction if len(sys.argv)==1: args={ "prototxt":"MobileNetSSD_deploy.prototxt.txt", "model":"MobileNetSSD_deploy.caffemodel", "confidence":0.2, } else: #lancement à partir du terminal #python3 ObjectRecognition.py --prototxt MobileNetSSD_deploy.prototxt.txt --model MobileNetSSD_deploy.caffemodel ap = argparse.ArgumentParser() ap.add_argument("-p", "--prototxt", required=True, help="path to Caffe 'deploy' prototxt file") ap.add_argument("-m", "--model", required=True, help="path to Caffe pre-trained model") ap.add_argument("-c", "--confidence", type=float, default=0.2, help="minimum probability to filter weak detections") args = vars(ap.parse_args()) # ModelNet SSD Object list init CLASSES = ["arriere-plan", "avion", "velo", "oiseau", "bateau", "bouteille", "autobus", "voiture", "chat", "chaise", "vache", "table", "chien", "cheval", "moto", "personne", "plante en pot", "mouton", "sofa", "train", "moniteur"] COLORS = np.random.uniform(0, 255, size=(len(CLASSES), 3)) # Load model file print("Load Neural Network...") net = cv2.dnn.readNetFromCaffe(args["prototxt"], args["model"]) if __name__ == '__main__': # Camera initialisation print("Start Camera...") vs = VideoStream(src=0, resolution=(1600, 1200)).start() #vs = VideoStream(usePiCamera=True, resolution=(1600, 1200)).start() #vc = cv2.VideoCapture('./img/Splash - 23011.mp4') #from video time.sleep(2.0) fps = FPS().start() #Main loop while True: # Get video sttream. max width 800 pixels frame = vs.read() #frame= cv2.imread('./img/two-boats.jpg') #from image file #ret, frame=vc.read() #from video or ip cam frame = imutils.resize(frame, width=800) # Create blob from image (h, w) = frame.shape[:2] blob = cv2.dnn.blobFromImage(cv2.resize(frame, (300, 300)), 0.007843, (300, 300), 127.5) # Feed input to neural network net.setInput(blob) detections = net.forward() # Detection loop for i in np.arange(0, detections.shape[2]): # Compute Object detection probability confidence = detections[0, 0, i, 2] # Suppress low probability if confidence > args["confidence"]: # Get index and position of detected object idx = int(detections[0, 0, i, 1]) box = detections[0, 0, i, 3:7] * np.array([w, h, w, h]) (startX, startY, endX, endY) = box.astype("int") # Create box and label label = "{}: {:.2f}%".format(CLASSES[idx], confidence * 100) cv2.rectangle(frame, (startX, startY), (endX, endY), COLORS[idx], 2) y = startY - 15 if startY - 15 > 15 else startY + 15 cv2.putText(frame, label, (startX, y), cv2.FONT_HERSHEY_SIMPLEX, 0.5, COLORS[idx], 2) # enregistrement de l'image détectée cv2.imwrite("detection.png", frame) # Show video frame cv2.imshow("Frame", frame) key = cv2.waitKey(1) & 0xFF # Exit script with letter q if key == ord("q"): break # FPS update fps.update() # Stops fps and display info fps.stop() print("[INFO] elapsed time: {:.2f}".format(fps.elapsed())) print("[INFO] approx. FPS: {:.2f}".format(fps.fps())) cv2.destroyAllWindows() vs.stop() vc.release()
Fontes de imagem para deteção de objectos
Pode utilizar este script com diferentes fontes de imagem. Para tal, é necessário adaptar ligeiramente o código anterior para modificar a variável “frame” que contém a imagem a analisar.
- A câmara Web do seu computador
vs = VideoStream(src=0, resolution=(1600, 1200)).start() while True: frame = vs.read()
- Uma câmara IP
vc = cv2.VideoCapture('rtsp://user:password@ipaddress:rtspPort') while True: ret, frame=vc.read() #from ip cam
- O Raspberry Pi Picam
vs = VideoStream(usePiCamera=True, resolution=(1600, 1200)).start() while True: frame = vs.read()
- Um ficheiro de vídeo
vc = cv2.VideoCapture('./img/Splash - 23011.mp4') #from video while True: ret, frame=vc.read() #from video
- Um ficheiro de imagem
frame= cv2.imread('./img/two-boats.jpg')
Resultados
Para este exemplo, enviamos uma imagem de dois barcos como entrada para a rede neural, que são corretamente reconhecidos. Para obter resultados ligeiramente diferentes, pode modificar o parâmetro de confiança para evitar falsos positivos.
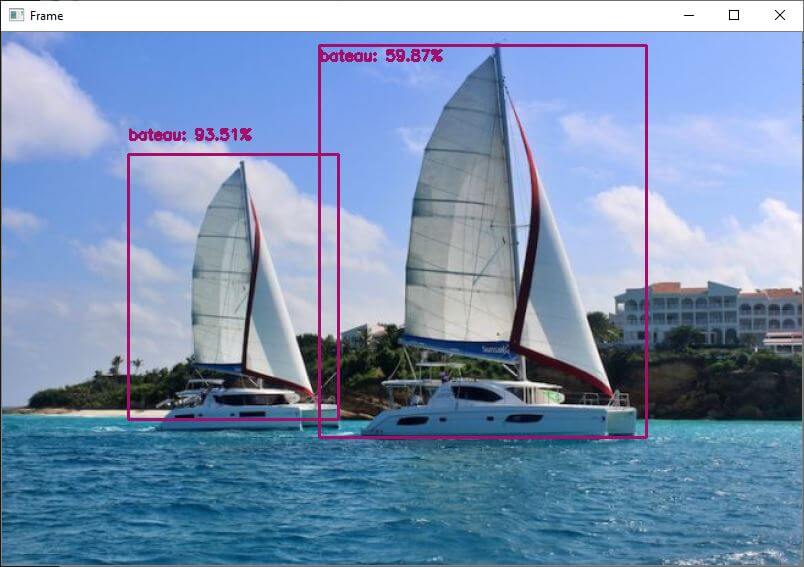
Pode testar este código com a sua webcam ou com fotografias, por exemplo, para ver o desempenho do modelo e do reconhecimento de objectos.
Pacotes e modelos
Neste tutorial, utilizámos o modelo ModelNet-SSD pré-treinado. Vale a pena notar que existem outros modelos de reconhecimento, como o Coco, e outras bibliotecas de reconhecimento visual, como a ImageIA.
Não hesite em deixar-nos um comentário para partilhar as ferramentas que utiliza ou de que tem conhecimento.