In this tutorial, we’ll train a MobileNetV2 TensorFlow model with Keras so that it can be applied to our problem. We’ll then be able to use it in real time to classify new images.
For this tutorial, we assume that you have followed the previous tutorials: using a TensorFlow model and preparing a database for training.
N.B.: I couldn’t find the right way to train the mobilenetV2 ssd model, as it is, with tensorflow. So I switched to Yolo. If you have the right method, don’t hesitate to leave a comment.
Recover an image database
Download one of the many image databases, such as cats-and-dogs, or create your own.
Unzipped the folder under Tensorflow>data
Model drive
To train the model, you can use the following script:
- load and expand the database
- create a model from the MobileNetV2 model(base_model)
- drive new model gains
- fine-tune base_model gains
import matplotlib.pyplot as plt import numpy as np import os import tensorflow as tf #_URL = 'https://storage.googleapis.com/mledu-datasets/cats_and_dogs_filtered.zip' #path_to_zip = tf.keras.utils.get_file('cats_and_dogs.zip', origin=_URL, extract=True) #PATH = os.path.join(os.path.dirname(path_to_zip), 'cats_and_dogs_filtered') PATH="./data/cats_and_dogs_filtered" train_dir = os.path.join(PATH, 'train') validation_dir = os.path.join(PATH, 'validation') BATCH_SIZE = 32 IMG_SIZE = (160, 160) #create train and validation sets train_dataset = tf.keras.utils.image_dataset_from_directory(train_dir, shuffle=True, batch_size=BATCH_SIZE, image_size=IMG_SIZE) validation_dataset = tf.keras.utils.image_dataset_from_directory(validation_dir, shuffle=True, batch_size=BATCH_SIZE, image_size=IMG_SIZE) class_names = train_dataset.class_names plt.figure(figsize=(10, 10)) for images, labels in train_dataset.take(1): for i in range(9): ax = plt.subplot(3, 3, i + 1) plt.imshow(images[i].numpy().astype("uint8")) plt.title(class_names[labels[i]]) plt.axis("off") val_batches = tf.data.experimental.cardinality(validation_dataset) test_dataset = validation_dataset.take(val_batches // 5) validation_dataset = validation_dataset.skip(val_batches // 5) print('Number of validation batches: %d' % tf.data.experimental.cardinality(validation_dataset)) print('Number of test batches: %d' % tf.data.experimental.cardinality(test_dataset)) #configure performance AUTOTUNE = tf.data.AUTOTUNE train_dataset = train_dataset.prefetch(buffer_size=AUTOTUNE) validation_dataset = validation_dataset.prefetch(buffer_size=AUTOTUNE) test_dataset = test_dataset.prefetch(buffer_size=AUTOTUNE) #augmented data (usefull for small data sets) data_augmentation = tf.keras.Sequential([ tf.keras.layers.RandomFlip('horizontal'), tf.keras.layers.RandomRotation(0.2), ]) for image, _ in train_dataset.take(1): plt.figure(figsize=(10, 10)) first_image = image[0] for i in range(9): ax = plt.subplot(3, 3, i + 1) augmented_image = data_augmentation(tf.expand_dims(first_image, 0)) plt.imshow(augmented_image[0] / 255) plt.axis('off') preprocess_input = tf.keras.applications.mobilenet_v2.preprocess_input rescale = tf.keras.layers.Rescaling(1./127.5, offset=-1) # Create the base model from the pre-trained model MobileNet V2 IMG_SHAPE = IMG_SIZE + (3,) base_model = tf.keras.applications.MobileNetV2(input_shape=IMG_SHAPE, include_top=False, weights='imagenet') #or load your own #base_model= tf.saved_model.load("./pretrained_models/ssd_mobilenet_v2_320x320_coco17_tpu-8/saved_model") image_batch, label_batch = next(iter(train_dataset)) feature_batch = base_model(image_batch) print(feature_batch.shape) base_model.trainable = False base_model.summary() #classification header global_average_layer = tf.keras.layers.GlobalAveragePooling2D() feature_batch_average = global_average_layer(feature_batch) print(feature_batch_average.shape) prediction_layer = tf.keras.layers.Dense(1) prediction_batch = prediction_layer(feature_batch_average) print(prediction_batch.shape) #create new neural network based on MobileNet inputs = tf.keras.Input(shape=(160, 160, 3)) x = data_augmentation(inputs) x = preprocess_input(x) x = base_model(x, training=False) x = global_average_layer(x) x = tf.keras.layers.Dropout(0.2)(x) outputs = prediction_layer(x) model = tf.keras.Model(inputs, outputs) base_learning_rate = 0.0001 model.compile(optimizer=tf.keras.optimizers.Adam(learning_rate=base_learning_rate), loss=tf.keras.losses.BinaryCrossentropy(from_logits=True), metrics=['accuracy']) initial_epochs = 10 loss0, accuracy0 = model.evaluate(validation_dataset) print("initial loss: {:.2f}".format(loss0)) print("initial accuracy: {:.2f}".format(accuracy0)) history = model.fit(train_dataset, epochs=initial_epochs, validation_data=validation_dataset) #plot learning curves acc = history.history['accuracy'] val_acc = history.history['val_accuracy'] loss = history.history['loss'] val_loss = history.history['val_loss'] plt.figure(figsize=(8, 8)) plt.subplot(2, 1, 1) plt.plot(acc, label='Training Accuracy') plt.plot(val_acc, label='Validation Accuracy') plt.legend(loc='lower right') plt.ylabel('Accuracy') plt.ylim([min(plt.ylim()),1]) plt.title('Training and Validation Accuracy') plt.subplot(2, 1, 2) plt.plot(loss, label='Training Loss') plt.plot(val_loss, label='Validation Loss') plt.legend(loc='upper right') plt.ylabel('Cross Entropy') plt.ylim([0,1.0]) plt.title('Training and Validation Loss') plt.xlabel('epoch') plt.show() #fine tuning base_model.trainable = True # Let's take a look to see how many layers are in the base model print("Number of layers in the base model: ", len(base_model.layers)) # Fine-tune from this layer onwards fine_tune_at = 100 # Freeze all the layers before the `fine_tune_at` layer for layer in base_model.layers[:fine_tune_at]: layer.trainable = False model.compile(loss=tf.keras.losses.BinaryCrossentropy(from_logits=True), optimizer = tf.keras.optimizers.RMSprop(learning_rate=base_learning_rate/10), metrics=['accuracy']) model.summary() fine_tune_epochs = 10 total_epochs = initial_epochs + fine_tune_epochs history_fine = model.fit(train_dataset, epochs=total_epochs, initial_epoch=history.epoch[-1], validation_data=validation_dataset) #plot fine learning curves acc += history_fine.history['accuracy'] val_acc += history_fine.history['val_accuracy'] loss += history_fine.history['loss'] val_loss += history_fine.history['val_loss'] plt.figure(figsize=(8, 8)) plt.subplot(2, 1, 1) plt.plot(acc, label='Training Accuracy') plt.plot(val_acc, label='Validation Accuracy') plt.ylim([0.8, 1]) plt.plot([initial_epochs-1,initial_epochs-1], plt.ylim(), label='Start Fine Tuning') plt.legend(loc='lower right') plt.title('Training and Validation Accuracy') plt.subplot(2, 1, 2) plt.plot(loss, label='Training Loss') plt.plot(val_loss, label='Validation Loss') plt.ylim([0, 1.0]) plt.plot([initial_epochs-1,initial_epochs-1], plt.ylim(), label='Start Fine Tuning') plt.legend(loc='upper right') plt.title('Training and Validation Loss') plt.xlabel('epoch') plt.show() #evaluate loss, accuracy = model.evaluate(test_dataset) print('Test accuracy :', accuracy) model.save('saved_models/my_model')
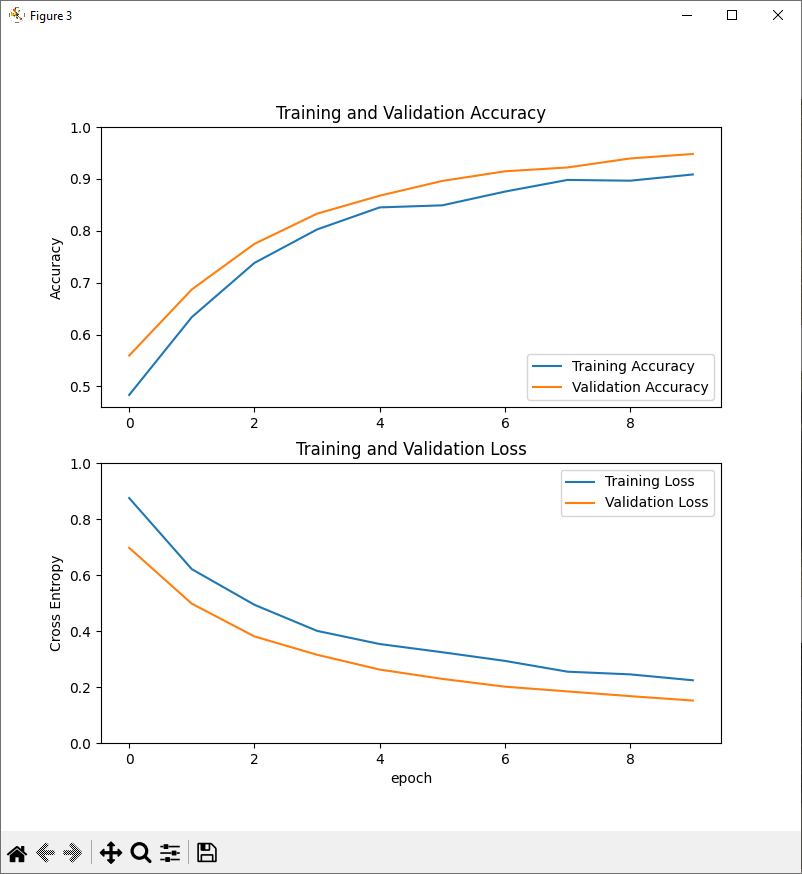
Using the trained model
You can use the trained model to classify new images containing a single object type per image. To do this, simply load the previously saved model (saved_models
#!/usr/bin/env python # -*- coding: utf-8 -*- # # ObjectRecognitionTFVideo.py # Description: # Use ModelNetV2-SSD model to detect objects on video # # www.aranacorp.com # import packages import sys from imutils.video import VideoStream from imutils.video import FPS import numpy as np import argparse import imutils import time import cv2 import tensorflow as tf from PIL import Image # load model from path #model= tf.saved_model.load("./pretrained_models/ssd_mobilenet_v2_320x320_coco17_tpu-8/saved_model") model= tf.saved_model.load("./saved_models/my_model") #model.summary() print("model loaded") #load class names #category_index = label_map_util.create_category_index_from_labelmap(PATH_TO_LABELS,use_display_name=True) def read_label_map(label_map_path): item_id = None item_name = None items = {} with open(label_map_path, "r") as file: for line in file: line.replace(" ", "") if line == "item{": pass elif line == "}": pass elif "id" in line: item_id = int(line.split(":", 1)[1].strip()) elif "display_name" in line: #elif "name" in line: item_name = line.split(":", 1)[1].replace("'", "").strip() if item_id is not None and item_name is not None: #items[item_name] = item_id items[item_id] = item_name item_id = None item_name = None return items #class_names=read_label_map("./pretrained_models/ssd_mobilenet_v2_320x320_coco17_tpu-8/mscoco_label_map.pbtxt") class_names = read_label_map("./saved_models/label_map.pbtxt") class_names = list(class_names.values()) #convert to list class_colors = np.random.uniform(0, 255, size=(len(class_names), 3)) print(class_names) if __name__ == '__main__': # Open image #img= cv2.imread('./data/cats_and_dogs_filtered/train/cats/cat.1.jpg') #from image file img= cv2.imread('./data/cats_and_dogs_filtered/train/dogs/dog.1.jpg') #from image file img = cv2.resize(img,(160,160)) img = cv2.cvtColor(img, cv2.COLOR_BGR2RGB) #input_tensor = np.expand_dims(img, 0) input_tensor = tf.convert_to_tensor(np.expand_dims(img, 0), dtype=tf.float32) # predict from model resp = model(input_tensor) print("resp: ",resp) score= tf.nn.sigmoid(resp).numpy()[0][0]*100 cls = int(score>0.5) print("classId: ",int(cls)) print("score: ",score) print("score: ",tf.nn.sigmoid(tf.nn.sigmoid(resp))) # write classname for bounding box cls=int(cls) #convert tensor to index label = "{}".format(class_names[cls]) img = cv2.resize(img,(640,640)) cv2.putText(img, label, (5, 20), cv2.FONT_HERSHEY_SIMPLEX, 0.5, class_colors[cls], 2) # Show frame cv2.imshow("Frame", img) cv2.waitKey(0)
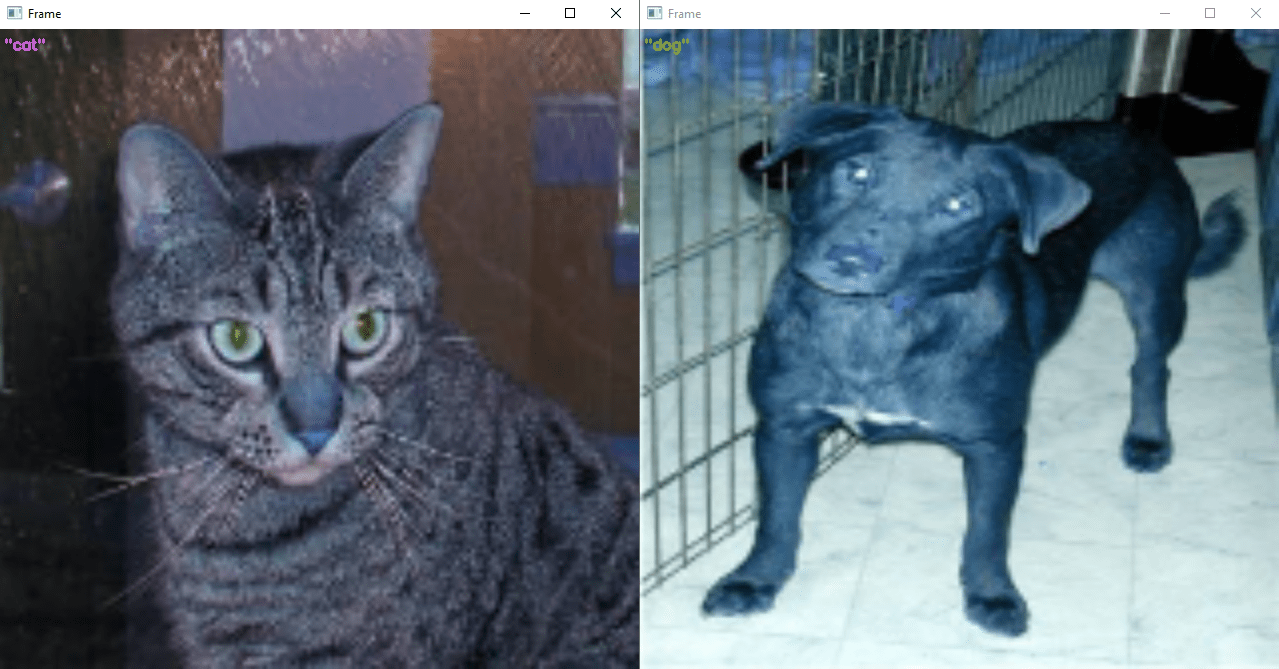
Applications
- recognition of different animal breeds
- recognition of different types of objects, such as electronic cards
Other classification models to consider
- vgg16
- vgg19
- resnet50
- resnet101
- resnet152
- densenet121
- densenet169
- densenet201
- inceptionresnetv2
- inceptionv3
- mobilenet
- mobilenetv2
- nasnetlarge
- nasnetmobile
- xception